Jim Simons: Quant King
When the Renaissance men stepped into the MF Global office in Hong Kong, it was a somewhat surreal moment. To say they stepped in could better be described as wafted or floated in, such was their calm demeanor. I couldn’t help noticing how relaxed they were and how super cool and confident. I don’t remember if it was Mark Rhoades-Brown or Gudjon Hermannsson or one of the others who wasn’t wearing socks, but it triggered a memory of the successful Goldman trader who used to sit on the end of a desk in London and who had dispensed with suit, tie, and shoes in preference for jeans and a T-shirt and grew his hair that little bit longer in a nonchalant, nonmaterial display of wealth and power—someone who was so self-assured and perhaps too successful to conform to normal dress habits and maybe also too important profitwise for management to question his comfortable attire. The Renaissance men had this aura. Why wouldn’t they?
We’d all heard of Renaissance Technologies of course—that hedge fund from East Setauket, New York, staffed with the smartest quants on Wall Street; the fund with one of the best winning runs in the markets that consistently delivered high double-digit, if not triple-digit, returns; the fund founded by a mercurial math professor and Cold War code breaker, Jim Simons. Our visitors had confidence in spades, the same assuredness in their abilities and system that you would attribute to John Henry. They were also backed up by a huge amount of capital. Now they were in our office and possibly about to give us a small piece of their secret. We were all ears!
Over dinner at the restaurant of the New York chef and Hong Kong gastro celebrity Harlan Goldstein in the International Finance Centre, the Renaissance men told us what they wanted: colocation of servers (in other words, proximity to the exchange) and also feeble commission charges for trade execution. Two essential elements to secure their trading edge. They were going to trade huge volumes! Speed was an essential element to complement their competitive advantage. How they would use that speed was open to debate and conjecture. They didn’t let on. After all, Renaissance was secretive in its affairs. Today you would guess it was a high-frequency trading strategy. Back in 2008, high-frequency trading was not nearly as prevalent. They wanted to eliminate erosion of profits due to excessive commissions. Their margins of profitability were obviously small. The quid pro quo would be high volumes. Our commission earnings would grow very quickly even though the cost per trade was tiny. Their profits would increase with the volume they could trade. If we could meet their demands, we might get a sniff of the action in executing their business.
ARBITRAGE
Speed is an essential element of most financial markets arbitrage: “The early bird catches the worm.” And arbitrage in the financial markets has been going on for years. Arbitrage in its basic form is the simultaneous buying and selling of the same product in two different venues to capture favorable price discrepancies. “Arbing” has become less crude and more refined nowadays as computers have displaced traders, but despite this, it still happens, and it is traded by many participants, in large amounts on a daily basis on the world’s markets and exchanges. Foreign exchange is one such market, and the volumes traded are colossal.
Arbitrage and trade have gone hand-in-hand for many centuries. Nowadays we choose to describe arbitrage as a simultaneous purchase and sale, but in many physical trading activities—that is, delivery of a physical good against payment—arbitrage historically involved a time lag between a purchase and sale. Arbitrage and trade were effectively interchangeable. Buying goods in one country, transporting them to another, and selling them for a profit would be considered trade hundreds of years ago; buying and selling that same good by telephone in the two different centers, having taken into account the transport costs, might well be classed as an arbitrage in modern times. Buying and selling an essentially similar good for a profit on two different exchanges by clicking on a computer mouse would be considered an arbitrage today.
The words “Silk Road” can evoke romantic visions of traders and merchants in times past, traversing vast wildernesses transporting goods from east to west, whether they be textiles, metals, or spices, and exchanging them for gold, silver, or other goods. Gold and silver were considered currency—a benchmark or measure of value. The price achieved for gold and silver could vary from country to country, and savvy merchants knew where to “arb” the precious metals—not without risks to their lives as there was often a high chance of ambush by bandits because not all routes were protected, and those that were required that a tax be paid for safe passage. Similarly, as bills of exchange (BOEs) evolved in Europe from the seventeenth century onward, an arbitrage evolved between the stated currency value on the bills of exchange and the price of its convertibility into physical gold and silver in two different geographical locations.
Arbitrage is present in all our lives. Banks wouldn’t exist without arbitrage. What banks pay out on savings and what they charge on loans is an arbitrage. If a mortgage lender engages in selling a fixed mortgage at the LIBOR, Euribor, SIBOR, or any other benchmark interest rate and the lender adds 0.5 or 1.0 percent or whatever else it can get away with, then consider yourself arbed by the mortgage lender. The spread over the benchmark is the lender’s arbitrage—that is, the lender’s profit. The lender can cover the trade far less expensively than the price you pay.
Arbitrage has many forms and guises. Some multinational companies go to great lengths to avoid paying taxes by booking profits in one location (where they pay less tax) and booking losses or booking smaller profits in another location (where they pay more tax). To elaborate on an example of a hypothetical tax arbitrage, let’s consider a company that has business interests in both Australia and Hong Kong. Let us assume a corporate tax rate in Australia of 28.5 percent and in Hong Kong of 16.5 percent, a difference of 12 percent, and for the sake of the example, a notional taxable profit of AUD$1 million is made in both centers after any taxable relief.
It could be argued that the company might pay AUD$120,000 less in taxes if its profits were booked in Hong Kong. A potential way to get around this tax burden might be to buy out-of-the-money FX option strangles in Australia and sell out-of-the-money FX option strangles in Hong Kong. In Australia, you would pay a premium to put this trade on and hope the trade expired worthless. In Hong Kong, the same option would expire worthless too, and you would collect the premium. This example is of course hypothetical, but it could happen in any two financial centers around the world. It would make even more sense if somehow the sold strangles made more premium than the bought strangles. This trade could be put on monthly to allow “time” to quickly work its decay on the option value. There may be subtle discrepancies in option prices on the exchange versus the OTC market. If the trades are booked as legitimate hedges in both centers, then the transfer of wealth is effectively made.
In the financial markets, some companies may headquarter or house subsidiaries of their businesses in locations where there is a less severe regulatory burden. Some might consider this regulatory arbitrage. It happens a lot in the foreign exchange markets. Generally these locations may require companies to pay up less capital and may well allow companies to offer excessive leverage to retail investors. Customers of course may choose where they trade, and it may well suit some customers to trade where they can obtain higher degrees of leverage. Less recognized regulatory centers are less likely to provide protective measures when it comes to the safety of client funds. That is the customers’ risk of course. In general, wherever there is a discrepancy, whether it be price, tax, or regulation, arbitrageurs will try to maximize their gain from the situation.
Modern-day arbitrage opportunities are traditionally found on futures exchanges where the same or very similar products are traded. A gold contract, for example, might be traded in ounces or in grams. There might be subtle differences in the quality of the gold—99.5 percent purity versus 99.99 percent. The contract might be traded in different currencies and countries. Each variable adds an opportunity for arbitrage—especially latency arbitrage! Trading on exchange versus over the counter also adds another variable. In commodities prices, there may be opportunities due to a market in contango or in backwardation. Contango is a situation in which the spot or cash price of a commodity is lower than the forward price. Backwardation is the opposite. It is a situation in which the spot or cash price of a commodity is higher than the forward price. The development of a backwardation is associated with supply shortage.
One other aspect of trading on exchange is that while there are a great many speculators, trend followers, and high-frequency traders now trading the markets, there are also bona fide hedgers. In perishable commodities, or in commodities that can be affected by the weather such as coffee, there will naturally be fluctuations in price that can lead to normal backwardation or contango markets. There is also the aspect of physical delivery. Add to the mix storage costs, transport costs, and interest rates, and soon the complexities exist to create enough potential distortions in pricing for sophisticated computer programs to juggle the numbers and arb the exchanges.
Exchanges thrive on volume, commissions, and clearing fees, and many new exchanges have popped up across the globe, more so since the explosion in high-frequency trading. If there is a genuinely liquid market for a product, an exchange (and an HFT) can quickly prosper. Not all contract specifications suit exchange participants, and some exchanges and their products have failed. Others have had teething problems when dealing with physical delivery.
In Oxfordshire, England, home of one of the oldest and great-est universities in the world, a farmer brought a nascent exchange to a standstill in an example of physical delivery arbitrage in which you have to admire him for his audacity and derring-do. His name was Clive Hawes, a giant of a man with an iron will to match. Hawes’s particular skill was that he was a livestock farmer and trader par excellence on the sheep and cattle markets. He had grown up as a boy learning from and watching his father, and he had taken over his father’s sheep flock, cattle herd, and other farming interests after his father’s untimely death.
At this stage as a 21-year-old, he was already importing and exporting cattle with his longtime school friend Ben Sparrow. While their career paths diverged soon afterward, Hawes and Sparrow would remain great friends until Hawes’s own untimely death at the age of 61. Hawes was never a man who did things in half measures, regularly buying vast amounts of livestock at Banbury Stockyard—reputedly Europe’s largest livestock market. In some years it would be sheep, but in 1990, his attention was drawn to an arbitrage on the recently created beef futures market. Hawes spotted that he could buy steers in the physical cattle market far cheaper than the price at which he could sell them on the futures exchange. And so he did. He bought pen after pen of steers, brought them home to correctly specified pens at his farm, and fed them appropriately to ensure that they reached the stipulated weight. A week before the contract was due to roll over, he instructed the exchange that he would deliver!
On a frosty winter morning, an impressive convoy of articulated lorries arrived at his farm, and several hundred cattle were loaded and sent to the abattoir. All was going to plan! But then of course a hitch arrived. The trader who had been buying the futures was none other than the owner of the abattoir—a burly man of rubicund complexion whose demeanor was changing by the second as lorry after lorry of braying steers arrived at his doorstep. As he fidgeted in his boots, his brow became increasingly furrowed, his fists clenched, and he reddened to the point of apoplexy.
The abattoir owner resorted to rejecting pens of cattle for the most obscure and tenuous reasons. He also refused to pay for the hides (or skins) of the slaughtered beasts, which would normally be the case. Tension mounted on both sides. While an abattoir in the middle of nowhere might have made an excellent location for a bare-knuckle fistfight, the two men were normally friends and well respected in the industry. The supervisors of the futures exchange were called in, and a gentlemen’s settlement was negotiated. The two men would laugh over it for many years. More important, they both learned lessons about risk!
Currency arbitrage generally happens between currencies traded on futures markets versus the OTC market. However, in the OTC market, subtle price discrepancies due to different quotes provided by genuine market makers can create moments when bid prices exceed offer prices, and in this area there is a phenomenal amount of what is called latency arbitrage. It must be said that brokers and platforms have mechanisms to avoid quoting inverse pricing, but trading companies that have multiple price feeds via an application programming interface can combine them into an aggregated price and then create a trading algorithm to capture any inverse prices and therefore capture free profits. (An application programming interface, or API, is defined as a set of functions and procedures that allow the creation of applications that access the features or data of an operating system, application, or other service.)
To illustrate a simple arbitrage in the spot market in euros versus U.S. dollars:
Provider 1 quote: EUR/USD 1.10101/1.10104
Provider 2 quote: EUR/USD 1.10105/1.10108
Provider 3 quote: EUR/USD 1.10107/1.10110
The best offer is 1.10104, and the best bid is 1.10107. The algorithm would sell the bid at 1.10107 and pay the offer at 1.10104. In this example, if the notional amount was EUR 1 million, the profit would be $30—(1 million × 0.00003). Prime brokerage and platform or API charges might add up to US$8 per million, or 2 million × 1.10, or roughly $17.6 for the trade. Profit works out at $12.4—not much, but if executed 1,000 times per day, it adds up to $12,400!
On January 4, 1999, the euro started trading on the over-the-counter market as the common currency of 11 countries. It wasn’t long before arbitrageurs noticed significant profitable opportunities in what became known as a triangular arbitrage between EUR/USD, USD/CHF, and EUR/CHF. The arbitrages were much larger than the fifth decimal arbs we see today. In early 2000, prices were quoted only to the fourth decimal. Societe Generale, a French bank, set up teams of arbitrageurs in London and New York to take advantage of the arbitrage. The requirements for inclusion in the teams were nimble fingers and the ability to handle quick mental arithmetic. For a period of time, especially the years from 2001 to 2005, Societe Generales’s profits were in the multimillions from this activity.
The arbitrage is slightly more complicated than the EUR/USD example, but it is easily explainable.
EUR/CHF is known as a cross-currency. It is quoted as EUR/CHF, or it can be created synthetically by combining EUR/USD and USD/CHF. For this example, I use fourth decimal quotes, typical of the early 2000s.
Say EUR/USD trades at 1.1010/1.1013 and USD/CHF trades at 1.0200/1.0205.
To buy EUR against Swiss francs, synthetically, one would first have to buy euros versus dollars (Buy EUR, Sell USD) and then buy U.S. dollars versus Swiss francs (Buy USD, Sell CHF).
So, to buy 1 million EUR/CHF, the following trades would need to be executed:
Buy 1 million EUR/USD at 1.1013 (it costs US$1.1013 million).
Buy 1 million, one hundred and one thousand, three hundred dollars against Swiss francs at 1.0205.
The cross-price is therefore 1.1013 × 1.0205, or 1.12387665 rounded up to 1.1239 to buy 1 million EUR/CHF. Similarly the bid price can be calculated as 1.1010 × 1.0200, or 1.12302 rounded down to 1.1230. Traders would most likely quote this pair at 1.1230/1.1240.
In the first instance, it seems highly unlikely that anybody could arbitrage a price as wide as 1.1230/1.1240, but as will become evident, a vast opportunity existed for this arbitrage, and the market lasted for several years. Societe Generale was not the only bank to profit from the arbitrage. Other traders at Bank of America and Fuji Bank were also most proficient at trading this particular triangular cross-trade. The trick was simple and something that Renaissance and others would copy and apply electronically to many other triangular cross-trades in the future. In the early 2000s it was sharp human minds, aided by real rather than artificial intelligence and by Microsoft Excel spreadsheets and two or three trading platforms, who executed the EUR/CHF arb in enormous quantities.
In reality, spot EUR/CHF did not trade at a 10 point spread. On the EBS platform, it might trade at 5 pips or even 3 pips, so the price seen by the traders might be 1.1235/1.1238. There were other factors in the market that helped give confidence in the trade, even if momentarily, one of the trading legs—there are three in a triangulation trade—was losing money relative to the spot price.
As an ex-Societe Generale trader explained, “Quite often you would have banks doing lots of Fix types of trades where they would come in and bid through the offer on the amount that was available on EBS. So, for example, on New York open a bank might receive an at-best order to buy 50 million EUR/CHF at market. The bank trader would basically bid through the offer. So say the market was 1.1210/13 in 3 million by 5 million. The bank might go 1.1214 bid for EUR $30 million. And he might execute the rest at the 1.1215 offer.
“On our side we had traders who could sense the weak side and so therefore might have offers in EBS for 5 million at 14 and 5 million at 15. Our trader would therefore be short 10 million EUR/CHF at the offer price. Eventually the market goes back into line, and during those times [the early 2000s], EUR/CHF would be in, say, a 30 pip trading range, so even if you were short at 17 in a 19 to 21 market, you knew you would have a chance to leg out at some point or the cross would come back lower.” He went on to say, “The bank trader would just jam the market because he had the at-best order. Regardless of how well he filled that order, he would just add a pip or two for himself and fill the client accordingly! So arbitrage was literally profiting from inefficiencies in the market.”1
“Arbing through the legs”—that is, creating a synthetic EUR/CHF buy trade to cover the short trade—was made easier by connecting price feeds in EUR/CHF, USD/CHF, and EUR/USD into an Excel spreadsheet. If the trader was indeed short at 17, he might be able to buy EUR/USD and buy USD/CHF and perhaps pay 15. Therefore making 2 pips. The traders would use a combination of EBS and Reuters platforms. In addition, they could call out to other counterparties to get quotes. Trading electronically was much faster, of course, and time was of the essence. So was saving commissions. On EBS, aggressors—or clients who “paid” offers or gave “bids”—would pay a commission, usually in the region of $20 per million U.S. dollars traded. Those who left resting orders would not pay the commission.
Traders typically had profit objectives of $2 million to $3 million annually with incremental bonus potential above those numbers. Some traders were not salaried and were just paid a percentage of performance. Interestingly, a lot of the traders were older, more experienced people. They were FX professionals who had been dealers for years and who had watched the ebb and flow and interaction of EUR/USD and USD/CHF through countless economic announcements over the years. They knew where the support and resistance were, had their ears to the ground for information, and were quick witted enough to instinctively feel when they were in profit or in loss. There was one trader who was so good he made in the region of $65,000 to $70,000 every day with just one or two trading losses per year. As his ex-colleague revealed, “Only when EBS allowed API trading did the machines take over. I never saw them perform like my friend. He was unbelievable. He saw moves before they happened. He smelled out the market.”
SIMONS THE MATHEMATICIAN
There are few in the market who have extrasensory perception—perhaps George Soros is one of them. And perhaps, in his capacity as an EUR/CHF arbitrage trader, the Societe Generale trader was another. Jim Simons, the founder of Renaissance Technologies, is most definitely in that league, and his credentials for sniffing out opportunities in the market, developing algorithms to do his sniffing for him, and capturing unbelievable profits are second to none.
Simons was not always a financial wizard. His background is in mathematics and academia. Born into a Jewish family in Brookline, Massachusetts, Simons excelled in mathematics and logic, often spending his nights thinking or “pondering” about solutions to problems, solving them, and then promptly forgetting them. He studied mathematics at the Massachusetts Institute of Technology and also at the University of California, Berkeley, where he gained a PhD at age 23. His pet subject was geometry, and along with Shiing-Shen Chern, he developed the Chern-Simons theory in secondary characteristic classes of 3-manifolds, a close relation of string theory. This concept is best described as the way in which point-like particles of particle physics are replaced by one-dimensional objects called strings and how these strings propagate through space and interact with each other.
After teaching at both Harvard and MIT, Simons moved to Princeton, where his love of geometry and skill at pattern recognition led him to work as a code breaker for the Institute of Defense Analyses albeit under the auspices of the National Security Agency (NSA). When he publicly contradicted his boss, retired four-star Army General Maxwell D. Taylor, in the New York Times Magazine over the merits of the Vietnam War, he soon found himself looking for alternative employment. And he found it at Stony Brook University on Long Island, where he took over as chairman of the math department in 1968.
Simons spent the next 10 or so years aggressively building up the department into a first-class operation. Along the way he would inspire and nurture a new generation of mathematicians, some of whom would eventually join him at Renaissance. He was recognized for his contribution to geometry in 1976, when he won the Veblen Prize of the American Mathematics Society for his work in this area.
One year later, in 1977, he set up Monemetrics, the precursor to Renaissance Technologies. His business partner was Lenny Baum, with whom he’d worked at the Institute of Defense Analyses. Baum was a cryptanalyst. An expert at code breaking, he could solve riddles of infinitesimal tininess in mammoth data streams, and, in theory, he and Simons would apply Baum’s algorithms to huge amounts of financial markets data in order to find hidden patterns and calculate the probability of their recurring. A small probabilistic edge would give them a statistical chance of beating the market! Two outstanding mathematicians—a geometer and a cryptanalyst—looking to crack the financial “Enigma code.”
THE MEDALLION FUND
In practice, Baum turned out to be a master fundamental trader and had little incentive to apply the algorithms he’d created. Enter James Ax, a probability theory expert who had worked for Simons at Stony Brook. Ax determined that Baum’s algorithms could be applied to securities, commodities, and currency markets, and so between Ax and Simons, they set up Axcom Ltd., an offshoot of Monemetrics that in due course would be renamed the Medallion Fund, at first a subscription fund but later the coveted employee-owned moneymaking machine that Renaissance Technologies is now so famous for.
Axcom’s double-digit profit making years abruptly stalled almost immediately after it was renamed Medallion. A nausea-inducing drawdown of 30 percent occurred from late 1988 until April 1989, testing the nerves and resolve of the two partners. Ax’s and Simons’s opinions diverged on how to deal with the problem, straining their working relationship to breaking point. Ax considered the drawdown acceptable within the model’s trading parameters and wanted to continue to trade. Simons wanted to stop, analyze, cogitate, and get to the bottom of the problems facing the fund. Both men remained resolute in defending their opinions, and so a standoff ensued. Elwyn Berlekamp, a fellow PhD and game theory expert at Berkeley who acted as advisor to Axcom, intervened and offered to buy out James Ax’s stake. The three men agreed. Ax left the fund, and Simons and Berlekamp worked out a way to get things back to profitability. It involved shortening the holding period of their trades—something that as the years passed developed into a much higher frequency trading strategy.
What was so powerful and different about Simons’s recruiting and trading approach compared to other funds was that he set about incrementally building up his business and its intellectual firepower by plucking the best brains he could find from the different fields of mathematics, physics, engineering, and computer science—himself, a geometer; Baum, a cryptanalyst; Ax, a probability expert; and Berlekamp, a game theorist. It was as if Simons was a modern-day John Galt. PhDs would be enticed to a utopian moneymaking phenomenon, each adding his or her particular skill and all working harmoniously under an avuncular visionary. Applying science to beat the markets was the name of the game. The people at Renaissance all added something to the mix, and just as he had done at Stony Brook with his math faculty, Simons kept building and adding different skill sets to keep his fund at the top of its game.
Aside from Ax, few Renaissance employees have voluntarily left the fund. There has been no seemingly sound reason to do so. Berlekamp is an exception; having brought the fund back to profitability and returning 55.9 percent (net of fees) in 1990, he also left. His desire was academia, and his passion was game theory, and he returned to UC Berkeley to teach math, where he remains today as professor emeritus. Money and the financial markets were seemingly less relevant to him. He found financial types “dull” compared to academics. It is a shame for Ax that he left under acrimonious circumstances and that he would not figure in the firm’s unparalleled successes over the next three decades.
THE RENAISSANCE TECHNOLOGIES PHILOSOPHY
Ax certainly had ideas, and for a while he made money using a relative value strategy. In the commodities markets, he would sell futures that had opened sharply higher and buy futures that had opened sharply lower from their previous day’s close, hoping to benefit from retracements to more normal levels. Simons alluded to employing the same tactics in stock picking when testifying to Congress after the global financial crisis in 2008. “Our trading models tend to be contrarian, buying stocks recently out of favor and selling those recently in favor,” he said. In a rare interview with Institutional Investor printed in November 2000, he explained his trading philosophy in a little more detail: “We look at anomalies that may be small in size and brief in time. We make our forecast. Then, shortly thereafter, we reevaluate the situation and revise our forecast and our portfolio. We do this all day long. We’re always in and out and out and in. So we’re dependent on activity to make money.”
The Renaissance philosophy is one of treating the financial markets as one giant experiment. The markets are in dynamic evolution. Simons’s quants or code breakers analyze any hypothesis and apply it to multiple markets. If technical analysts like Edwards and Magee look for patterns, then Simons and his analysts look for patterns within patterns. He has said, “We search through historical data looking for anomalous patterns that we would not expect to occur at random. Once we find one, we test it for statistical significance and consistency over time. After we determine its validity, we ask, ‘Does this correspond to some aspect of behavior that seems reasonable?’
“Many of the anomalies we initially exploited are intact, though they have weakened some. What you need to do is pile them up. You need to build a system that is layered and layered. And with each new idea, you have to determine, is this really new, or is this somehow embedded in what we’ve done already? So you use statistical tests to determine that, yes, a new discovery is really a new discovery. OK, now how does it fit in? What’s the right weighting to put in? And finally you make an improvement. Then you layer in another one. And another one.”2
The quest is to beat the markets because in the view of Simons and his analysts, the markets are still inefficient, although Simons contends that the markets are “considerably more efficient” and that “efficient market theory is correct in that there are no gross inefficiencies.”3 About a third of Renaissance’s 275 staff members hold PhDs in all manner of disciplines. Bob Mercer and Peter Brown, who both joined Renaissance in 1993, are the founders of a speech recognition group for IBM. Gudjon Hermannsson has a PhD in computer science from Stony Brook. Mark Rhoades-Brown, himself a nuclear theorist by training, pointed out at the University of Tennessee, Knoxville, Graduate Awards Ceremony in 2002 that the biggest chunk of Renaissance’s scientists are physicists. He also said that the value of critical thinking is as an asset that many scientists possess and one “that has value in the outside world beyond physics.” In quantum physics, small causes can have large effects. It is known as the butterfly effect, and it forms part of chaos theory. If any hedge fund can make sense out of chaos, it is Renaissance. In the quant world, everything is relevant!
Such is the culture at Renaissance that freedom of expression is paramount on the totem pole of values; critical thinking and problem solving are passions, and moneymaking is an inevitable side reward. The people at Renaissance are living the American dream in real time and using their brainpower and brilliance to continuously beat the market. Their results of the last 28 years are testament to the fact the markets can be beaten and also that one must evolve with the market to keep winning. One can expect that with the caliber of personnel that they employ and the techniques that they utilize, Renaissance will stay at the top of the pile for many years to come.
THE MEDALLION FUND UNDER MERCER AND BROWN
Simons stepped aside from leading Renaissance Technologies at the end of 2009, handing the reins to Bob Mercer and Peter Brown, who were by then 16-year veterans of the company. Their privilege was to take over the Medallion Fund with average annual returns above 40 percent with only one losing year in 1989. Simons, who had set up Math for America in 2004, whose mission is to improve mathematics education in the U.S. public schools, would concentrate more on his philanthropic activities. By 2009, Renaissance was managing other funds, including the Renaissance Institutional Equities Fund and two “diversified alpha” funds. Its assets under management were north of $20 billion.
The question that would be asked was whether Brown and Mercer could continue making stellar returns. The answer was a resounding yes, certainly for their in-house fund, as Medallion posted a 33.9 percent return in 2010 (Table 6.1).
TABLE 6.1
Renaissance Medallion Fund Returns for 1990 Through 2016
Renaissance Medallion Fund Returns for 1990 Through 2016
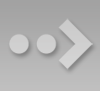
In the 27-year period from 1990 to 2016, Renaissance had turned $1,000 in its Medallion Fund into over $13.2 million. And this was after fees, which ramped up over the years to a 5 percent management fee and a 44 percent performance fee. It is quite the most remarkable performance record of any fund, and it also shows how compound interest works in the most extraordinary way.
Mercer and Brown would increasingly focus on high-frequency trading, and along with other hedge fund giants such as Ken Griffin’s Citadel, they would extract profits not just by sniffing out patterns but by employing powerful computers to provide split-second liquidity to any manner of markets and then just as rapidly off-loading any positions that might be accumulated straight back to the market. Their split-second intervention, often using large amounts of leverage, would collect small favorable price discrepancies in what amounted to a large-scale arbitrage operation.
HIGH-FREQUENCY TRADERS
In the currency markets, other HFTs have become very well known, certainly among brokers and electronic communication networks. Names that keep cropping up are Lucid Markets, GSA Capital, and Virtu Financial. All have pretty much the same modus operandi and similarly describe their “liquidity provision” in glowing, nonpredatory terms.
Virtu, a company listed on Nasdaq, in its company overview says, “Virtu’s liquidity provision plays a vital role in the overall health and efficiency of the global financial markets, especially in times of market turbulence.” And it also says, “As market makers, we lower costs for both retail and institutional investors by supplying competitive bids and offers, without seeking to take on risky directional positions.”
Lucid Markets, owned by FXCM and founded by ex-Goldman Sachs alumni Mat Wilhelm and Dierk Reuter, is “focused on delivering a compelling liquidity offering for FX markets accessible via API.” Reuter, incidentally, is a former global head of algorithmic trading at Deutsche Bank, and he holds a PhD in aerospace engineering from the Georgia Institute of Technology.
Founded in 2005 by Oxford University math graduate Jonathan Hiscock, who is a former managing director of statistical arbitrage at Deutsche Bank, GSA Capital is somewhat more transparent, if only insofar as the company admits to being a prop shop. “We run a number of absolute return, alternative strategies focused on systematic trading across liquid equities, futures, and foreign exchange markets globally.” They do this on a “world-class” proprietary platform and trade on “multiple time frames.”4
SPINNING THE STACK
Collectively, these non-bank market makers have challenged the more traditional liquidity sources provided by giants in the FX market such as UBS and Deutsche Bank, but at the same time they have incurred the wrath of some less adept providers by continually picking them off in a game of cat-and-mouse arbitrage. Some banks have complained that HFTs have been “spinning the stack” in aggregated liquidity pools. The concept is not too dissimilar to “whale order hunting” in equity markets.
An example of a liquidity stack is shown in Figure 6.1. This is an aggregated stack of liquidity in USD/JPY. Anybody wishing to buy $40 million would be able to do so theoretically at no worse than 118.03. The volume-weighted average price (VWAP) is actually 118.0238. (To get this, you multiply volume by price for all the amounts offered to achieve an average price, which in this case was $40 million.)

Figure 6.1 Liquidity stack, USD/JPY.
HFTs scrabble to be top of book—that is, to be the best bid and offer, or 118.01/118.012. And they will trade against small orders (and each other) to maintain the top-of-book position. If the 118.012 offer is taken out by a large order, the HFT is quick enough to buy at 118.014 in front of the big order and resell at a higher price. Say the order is for $40 million. The price might get distorted as shown in Figure 6.2. An HFT “spins the stack” to its advantage.
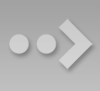
Figure 6.2 Liquidity stack with HFT intervention.
The new VWAP for $40 million is 118.0314 compared to 118.0238. A profit of 0.0076 pip in $40 million. This amounts to 304,000 yen, or $2,575.4 (marked-to-market at 118.04). Far from delivering a compelling alternative liquidity source, the HFTs have just imposed a rather noxious trading tax.
THE PROFITABILITY OF HFTS
In the year ended December 2011, Lucid, whose focus was entirely on FX, made operational profits of $113.4 million on $13.4 trillion of volume using its market maker/taker model. GSA Capital split profits from its multiple asset strategies of GBP 71.4 million among its partners for the period up to March 2013. Virtu reported that 20 percent of its $414.5 adjusted net profits for the year ending December 31, 2013, or about $82.9 million, came from FX. It was a maker/taker on the following platforms: CME, ICE, Currenex, EBS, Hotspot, Reuters, FXall, and LMAX. Virtu listed on Nasdaq on April 15, 2015, raising $314 million from its IPO and valuing the company at over $2.5 billion.
In the high-frequency game, Renaissance’s Medallion kept returning profits of 20 percent plus, but some of its other funds were posting distinctly mediocre returns. In 2012 Renaissance Institutional Equities Fund posted profits of 8.43 percent, and Renaissance Institutional Futures Fund (RIFF) actually lost 3.17 percent. In October 2015, Renaissance announced it would close RIFF, citing a lack of investor interest. The reality was that the fund was a relative underperformer, posting average annualized returns of 2.86 percent (after fees) since its inception in September 2007. A fund holding about $1.46 billion in 2013, it posted profits of just 2.15 percent, or about $31 million. Compare this to Virtu’s roughly $95 million from Global Commodities in 2013 (Global Commodities’ trading accounted for 23 percent of Virtu’s adjusted net earnings of $414.5 million), and it would seem that Renaissance had either at least partly lost the battle in latency arbitrage or had an issue with its algos. Other viable explanations are that the market had reached saturation levels with many HFTs employing the same strategies or that markets had indeed become efficient and arbitrageurs had served their purpose.
It’s interesting to read Virtu’s stated “competitive strengths” in its prospectus summary:
1. Critical component of an efficient market ecosystem
2. Cutting-edge, proprietary technology
3. Consistent, diversified, and growing revenue base
4. Low costs and large economies of scale
5. Real-time risk management
6. Proven and talented management team5
It would seem that points 2 and 4 are definitely competitive advantages. Scale and low costs are highly beneficial to making outsize profits. Virtu has had only one losing day in over 1,200 trading days. Their proprietary software is working for now. Point 3 is debatable: other companies such as Renaissance prefer to concentrate on stocks, bonds, commodities, and currencies that are publicly traded, liquid, and amenable to modeling. So diversification into non-liquid investments has plenty of associated risks.
Thankfully, Virtu has “real-time risk management”—a must have for the frequency of the company’s trading. There will be plenty of discussion about point 1. In some respects, HFTs make for an efficient market ecosystem, but only in very small trading amounts. Pension funds might well grumble that order execution is far more difficult and less efficient due to the many thousands of orders that flurry through the system the instant they try to execute a large trade. One would hope that Virtu’s management team is proven and talented. It certainly seems to be: $414.5 million of net adjusted profits in 2013 is an enormous amount of money.
But what of the weaknesses that could hinder profitability? These would apply to Renaissance, Citadel, GSA, Lucid, or any other HFT:
1. Because our revenues and profitability depend on trading volume and volatility in the markets in which we operate, they are subject to factors beyond our control, are prone to significant fluctuations, and are difficult to predict.
2. We are dependent upon our trading counterparties and clearing houses to perform their obligations to us.
3. We may incur losses in our market making activities in the event of failures of our customized trading platform.
4. We may incur material trading losses from our market making activities.
5. We are exposed to losses due to lack of perfect information.
6. We face competition in our market making activities.
7. We are subject to liquidity risk in our operations.
8. Rules governing designated market makers may require us to make unprofitable trades or prevent us from making profitable trades from time to time.
9. Regulatory and legal uncertainties could harm our business.6
There’s plenty to sift through here, but most glaring are volume, volatility, liquidity, competition, and regulators. Much to the chagrin of many HFTs and some exchanges, there have been several recent books that expose some of the moneymaking methods of HFTs. In addition, a new phenomenon in the markets known as “flash crashes” seems to be a constant risk to stability and order in the markets—the blame for which some have placed squarely at the door of HFTs. The begging questions are whether HFTs actually add value in the sense of “liquidity provision” and whether their outsize profits are genuinely sustainable. In the long run, markets become efficient because arbitrageurs iron out inefficiencies. A hotter topic, and one that will undoubtedly cause debate and perhaps uproar, is the extent to which retirees suffer in their pension schemes due to market slippage.
SMALL AND MEDIUM-SIZED ARBITRAGE TRADING FIRMS
It would seem pretty daunting for anyone, especially a layman foreign exchange trader, to even contemplate arbitrage trading, and yet it is possible—no PhD required! To trade in anywhere near the scale of a Virtu or a Renaissance firm would require a huge capital outlay, investment in infrastructure, technology, and key personnel. But even faced with such daunting competition, smaller traders with normal accounts can make money using an arbitrage strategy without eating into their life savings. How is this possible? A small capital investment will not lead to billions in profits, but it can produce positive, relatively risk-free returns—something that no other single FX strategy can match.
The key is to trade products that are less liquid and are found in more remote parts of the world. Somewhere outside the reach of algorithmic HFT behemoths. Trading new products on fledgling exchanges against established OTC products can lead to potential arbitrage opportunities. Triangular trades in different centers add complexity, as does trading longer time frames. Increased leverage magnifies small gains into acceptable returns. In using arbitrage strategies, it is essential to keep trading and borrowing costs to a minimum. It is also a prerequisite to use a broker or bank that is well capitalized and has a stable balance sheet.
In recent years Dubai and Abu Dhabi have emerged as alternative trading centers that span the crossover between Asian markets and London. ADS Securities in Abu Dhabi is the region’s largest regulated retail FX broker. Dubai, originally known for oil production and as a center for international trade, has emerged as an incredible twenty-first-century city, with excellent infrastructure, a superlative airline, outstanding architecture, and unbeatable facilities. During this time, it has welcomed many new inhabitants from many cultures while maintaining its own heritage, dignity, and traditions.
Dubai is a city that has embraced change, encouraged technology, and promoted entrepreneurialism, new business enterprises, and commerce. In the financial services sector it now ranks as a respected alternative for the establishment of hedge funds or indeed foreign exchange brokerages, under the auspices of the Dubai International Finance Authority. Its futures exchange, the aptly named Dubai Gold and Commodities Exchange (DGCX), has seen 10 years of impressive growth as the premier futures exchange (outside of India) for trading Indian rupees and now other less readily traded currencies such as the Russian ruble, Chinese renminbi (yuan), and South African rand.
NON-DELIVERABLE FORWARDS (NDFS)
The DGCX commenced trading in November 2005, and it launched the world’s first Indian rupee (INR) futures contract in 2007. Beginning in 2010, the DGCX started to see unprecedented levels of volume as arbitrage opportunities arose on contracts traded on DGCX INR futures versus non-deliverable forwards (NDFs) offered by banks and accessed through FX brokers. In Dubai there was a natural requirement for trade in Indian rupees because a large proportion of Dubai’s 2.5 million inhabitants hail from India. There are many merchants and business owners who are non-resident Indians. The DGCX formed a natural exchange for the many businesses located in Dubai that wanted to transact “on exchange” in Indian rupees.
As the market developed, slight disparities appeared between locally traded Indian rupees on the DGCX and the more globally traded non-deliverable forwards offered by many banks with a presence both in India and outside of India. Banks such as Deutsche Bank, Standard Chartered Bank, HSBC, and many others have a presence in India. They are also providers of an NDF outside of India. Non-deliverable forwards allow for trading in currencies such as the Indian rupee, Chinese renminbi (yuan)(CNY), Brazilian real (BRL), and South Korean won (KRW), among others, outside of these countries. Settled in dollars, the local currencies are never delivered. The conversion rate—known as “the Fix”—for both onshore and offshore markets in the Indian rupee is set daily by the Reserve Bank of India (RBI).
Globally, NDF volumes remain surprisingly small, with estimates ranging for daily turnover of $17 billion in INR and $19 billion in KRW, with $16 billion in BRL and CNY trading up to $17 billion per day. The Bank of International Settlements Triennial Survey of 2013 estimated that the total daily turnover for the whole NDF market came in at a mere $125 billion. The populations of these countries—as well as Russia, Malaysia, Indonesia, Colombia, Taiwan, and the Philippines—whose currencies can be traded offshore as NDFs, far exceed those of the G10 countries, yet offshore turnover in their currencies accounts for only 2 percent of the whole. The non-deliverable option (NDO) market is even less sophisticated and represents a huge potential market. NDF trading is largely unchartered territory with massive potential both for growth and for arbitrage trading. The fact that the DGCX now offers contracts in Russian rubles and Chinese renminbi (yuan) presents undoubted opportunities.
The Indian rupee arbitrage really gathered momentum from the years 2011 to 2014. In Dubai, the exchange-traded U.S. dollar price would tend to open up bid versus the rupee and relative to the NDF price, especially in the early part of the month. The price on the DGCX more or less mirrored the onshore price on the Indian MCX exchange, reflecting demand for U.S. dollars to buy, among other things, oil. The NDF price in USD/INR was affected by a whole basket of different currency interactions and often lagged the pure onshore price. Indian rupee forwards were calculated to mirror the impressive interest rate “carry” in India, which represented attractive yields for those offshore willing to accept exchange risk.
In many instances the dollar was sold offshore as speculators accumulated rupees. The arbitrage, therefore, was to buy U.S. dollars in the NDF and sell U.S. dollars on the futures exchange. In periods of rupee strength, as investment in India and demand for rupees accelerated, carry trading became doubly attractive as traders were paid interest to hold on to rupees and also benefited from an appreciating currency. Almost in tandem, arbitrage opportunities came around much more frequently. And virtually on a daily basis the arbitrage would fluctuate between 2 and 5 pips and sometimes reach 7 pips. On rare occasions it could reach 15 or more pips.
To work out the profit on a USD$1 million position capturing 5 pips, an example calculation is as follows:
Buy $1 million USD/INR one month forward at 65.00.
Sell $1 million USD/INR one month forward at 65.05.
Profit = 0.05 × 1 million, or 50,000 rupees. Marked-to-market at 65.00 = 50,000/65, or $769.23.
Brokers typically offered 3 percent margin or 33.33 times leverage. So in order to put on a $1 million position, it would take just $30,000 in capital. Multiply this by 2 because two positions (a buy and a sell) were involved to lock in the profit one month forward, and the monthly return on $60,000 was an impressive 1.282 percent, or over 15 percent per year. In reality traders made much more as the arbitrage would tend to from 5 pips to zero as the month end approached and might even reverse. Positions could thus be unwound and put on in the next calendar contract. The opportunities were vast, and the trades could be done with the click of a mouse. And what’s more, the trade was open to anybody who could access the DGCX and offshore NDF pricing. And it still is.
LIMITATIONS ON TRADING NDFS
There were some limitations to the trade, scale being one of them. Many brokers had concentration risk limits set by their prime brokers. These tended to be in the area of a $300 million to $500 million net open position. There were only seven or eight brokers offering the NDF, and these tended to use the same prime brokers. As the arbitrage generally favored being long U.S. dollars in the NDF, and since brokers passed the positions via a straight-through process to prime brokers, brokers were left holding large quantities of short U.S. dollar positions at prime brokers such as UBS, Deutsche, and Citibank.
Other limitations to the trade were the brokers themselves. In 2011, MF Global collapsed, and many of its clients trading NDFs had difficulty retrieving their funds during the bankruptcy proceedings. In September 2014, Marex Spectron confirmed that it was to close its FX business, and in May 2015, Fixi Plc ceased to offer NDF trading. One client of Refco and then MF Global has warned investors that it is imperative that they find a stable broker. The ability to withdraw funds in a timely manner is essential if you are trading with high degrees of leverage and at several brokers. Profits from one broker have often been withdrawn and used to meet margin calls at another broker. Any disruption to the flow of funds can mean trades getting closed out—a risk in itself because the other side of the trade remains open and to close that out too means paying away the spread. But finding funds to trade when your own legitimate funds are tied up elsewhere through no fault of your own is extremely galling. Brokers going bankrupt only ramps up the stress.
There is in an old saying in the financial markets: “Nothing kills success like success.” It is true that its success in Dubai led other exchanges to try to offer an Indian rupee contract. In December 2012, the CME launched an Indian rupee futures contract, followed in November 2013 by Singapore’s SGX. Some CME salespeople made a habit of touting the arbitrage opportunities as a fait accompli and reason to trade Indian rupee futures, and sure enough, new participants including high-frequency trader Indian rupee market maker/takers joined the party. The arbitrage subsequently virtually disappeared. A new saying that might be coined is “Nothing kills a successful arbitrage like an HFT,” and yet from time to time, certainly in Dubai, where there is a natural flow of Indian rupees and genuine market hedgers on exchange, there still exist opportunities to arbitrage. And in the cyclical ebb and flow of the markets, that arbitrage may come back in reasonable proportions when the dollar weakens!
One hardened and successful arbitrage trader has reiterated that the stability of counterparties is a prerequisite in trading arbitrage, especially if you are to put down significant amounts of capital. Safety of client funds is paramount! A recent arbitrage in Australian dollars on exchange versus OTC illustrates this point.
In the burgeoning market of retail foreign exchange, many incentives are offered to entice new traders to start trading, one of which may be on rollover costs. If a client holds a currency that pays interest, he should receive interest on his position. The counter is true also. If he is short that currency, he should pay interest. Retail brokers who expect their clients to “blow up” sometimes offer a loss leader and might not charge interest on short positions. The Australian dollar is a good illustration of this. The Reserve Bank of Australia (RBA) may, for example, hold interest rates at 2 percent. One would reasonably expect to earn 2 percent annually for holding Aussie dollars and pay 2 percent annually for borrowing them. Naturally if a counterparty doesn’t charge interest on short positions, if you can find one that does pay interest on long positions, you have an arbitrage. Thus 2 percent multiplied by leverage of, say, 50 times makes for a 50 percent annual return (100 percent/2 as there are two trades: one at the broker and one at the futures exchange). One such arbitrage arose between a broker in a less recognized jurisdiction and the CME.
Here’s the trade:
Sell AUD spot at the offshore broker. Let’s say 0.7268.
Buy three-month future, say, 0.7238.
Arbitrage is 30 pips, or US$3,000 per AUD million.
The profit is locked in.
There are a couple of issues for the retail broker here. First, he is expecting his clients to overtrade, or he is expecting trades on the long side to cover the client short positions. If he is running a B Book, his profits magnify as trades net off. What the B Book doesn’t want is to be on the other side of a large short AUD trade in a market where the AUD is weakening. Unfortunately for the broker, this is precisely what happened. If a short 100 million AUD/USD position moves 100 pips lower, then the B Book will lose $1 million. If the broker is capitalized at less than $1 million, then all things being equal, he is insolvent.
In the period from October 2014 to October 2015, the AUD dropped from 0.89 to 0.69. The arbitrage worked for sure. The broker was not as successful.
The advice from the arbitrager, who luckily got his capital back but whose broker reneged on the free rollover, causing him to lose money, is as follows: “There will always be arbitrages, but make sure they are genuine and accessible to everyone. Your returns might not be so large, but you won’t lose your capital.” He goes on to say, “A lot of products seem genuine, but they are not. Avoid lucrative no-brainer trades, and ask yourself the questions ‘How come?’ and ‘Why?’ You will save yourself a lot of pain later. Above all, you must protect your capital. And that can only happen if you select your counterparties who are appropriately regulated and have correct protection of client funds.”7
THE MONEYMAKING POTENTIAL IN ARBITRAGE
Making money can be a tremendously exciting business. And making tons of money for free is every arbitrageur’s dream. There are plenty of success stories of smaller-scale arbitrageurs who have made many thousands if not millions of dollars. What unites all of them is that they tend not to shout about their moneymaking edge. Arbitrage is the business of making money from inefficiencies in the market. It makes no sense whatsoever to share that competitive advantage because doing so limits the life span of the trade. There will always be arbitrages across markets and exchanges, and savvy investors can profit from being alert and prepared.
On a grander scale, the progress in computer technology and wizardry has meant that a few cutting-edge super quants with powerful computers, excellent communication networks, and proximity to pricing servers have evolved as giant moneymaking machines.
With respect to Renaissance, while secrecy is paramount, it also has super brain power in the sciences as one of its competitive advantages. Under its roof, it accommodates PhD scientists in math, physics, aeronautical engineering, and computer science, among others. Brilliant people who can spot patterns in charts before they become patterns, who study speech, and who model behavior. Sure, they have gone down the HFT route, but they had the scale to invest in infrastructure and the ability to apply their models over ever diminishing time frames.
AN INSPIRING LEGACY
Jim Simons is surely one of the smartest people ever to have traded the markets. Over a 20-year period, his returns were astronomical, and he built a phenomenal legacy to hand off. His Renaissance fund is among the most successful funds ever launched, and it is the beacon for professionalism and performance. His staff members have been rewarded for being critical and creative and for challenging the norms. They have mostly made a fortune from trading stocks, bonds, currencies, and commodities that are “liquid, publicly traded, and amenable to modeling.” If there are indeed patterns in the market, Renaissance has discovered them, which can give hope to any budding trend follower, although Simons has observed that “efficient market theory is correct in that there are no gross inefficiencies.”8
You cannot help but like and admire Jim Simons. He’s done his bit for his country as a Cold War code breaker. He created an outstanding mathematics faculty at Stony Brook University. He has shown the world of finance that you can beat the markets, and he now acts in a philanthropic capacity promoting and sponsoring Math for America. Among the quants, he is the best of the best, and he has created the same in Renaissance Technologies. He is a financial markets genius and stands without equal as the ultimate Quant King.
Unnamed Societe Generale trader, personal interview with Ben Robson, 2014.
2. Hal Lux, “The Secret World of Jim Simons,” Institutional Investor, November 1, 2000.
3. Ibid.
5. Virtu Financial, Inc., Form S-1 filed with the Securities and Exchange Commission, March 10, 2014, https://www.sec.gov/Archives/edgar/data/1592386/000104746914002070/a2218589zs-1.htm.
6. Ibid.
7. Rakesh Daryani, personal interview with Ben Robson, 2014.
8. Hal Lux, “The Secret World of Jim Simons.”
Renat Fatkhullin: Social Trading and MT4
If Mark Zuckerberg and Renat Fatkhullin were standing side by side in a taxi stand, a few heads might turn. Most people would instantly recognize Zuckerberg. “He’s that guy from Facebook!” or “It’s that social media guy,” some might say. “Why’s he waiting for a taxi? He’s worth billions. Who’s that person he’s standing next to?” It would be hardly surprising that anybody outside of the computer programming world or very few inside or outside of FX would not recognize Fatkhullin.
A man more secretive than option trading Swiss billionaire Urs Schwarzenbach, Fatkhullin is to social trading as Zuckerberg is to social media. His products are so sought after that they are a must have for almost every currently operating retail FX and CFD brokerage. Several companies have tried to issue an IPO on the back of “their” client bases using “his” software. The scale and reach of his platform and associated products is unsurpassed by any of his FX market making contemporaries. He does not have many serious competitors, such is the demand of brokers in the retail space to license his software. He remains humble, down to earth, and reachable by like-minded technology and computer individuals. He doesn’t boast of his wealth and remains selfless. No “rich lists” for him, although if he were in them, he would surely be near the top.
His company is MetaQuotes; his trading platform is MetaTrader. It is the single most popular and successful trading platform ever to reach the market. If there were one person who symbolized everything to do with the success of retail FX and CFD trading, it would be Renat Fatkhullin, and yet he is neither a trader nor a market maker. He is, rather, an enabler. He has enabled brokerages to offer a low-cost scalable platform to their client bases. He has adapted his platform for ease of use on mobile and Android devices. He has created a programming language that allows for Expert Advisors and robots to provide either trading signals or algorithmic trading. His platform has charts, allows for up-to-date news, and is user-friendly. He fills the need for clients new to trading to trade in very small sizes or utilize many of the “expert” tools provided side by side on iterations of his platform. His software allows brokers to manage the risk that they take on by being counterparty to trades or pass it on to their banks. In short, he offers many things to many people. In the process, he has become a billionaire. He has achieved this in a little under 16 years.
Fatkhullin’s success story initially ran in tandem with that of Alpari, the now defunct London-based FX and CFD broker. While Alpari was formed on Christmas Eve 1998, it wasn’t until November 2000 that MetaQuotes Software Corp. was established. In what the Alpari founder and chair, Andrey Dashin, has described as a “historic” meeting with Renat Fatkhullin, in that same month, Alpari would take its trading online using the newly developed MetaQuotes platform. Retail FX trading was in its nascence with as yet no broker or platform dominating the market. Alpari would join the likes of CMC Markets, FXCM, CMS, Saxo Bank, and GAIN Capital and would initially offer online trading in 11 currency pairs.
Apart from envisioning the most outstanding opportunities of scale and profits, many of these companies had other common similarities, not the least of which was a connection with Russian computer programming engineers. There are many schools in Russia that offer computer science and information technology degrees, and for some reason, there seem to be a great many Russian computer engineers and programmers working in the field of FX—and nowadays, more and more in the fields of algorithmic and high-frequency trading. Perhaps a few were inspired by George Soros, who donated many tens of millions to projects in Russia as part of his efforts as a philanthropist and whose George Soros Foundation constructed 32 computer centers at universities in Russia’s major cities.
In an interview with FX-MM magazine, Denis Sukhotin, Russian founder of FxPro, says of his desire to succeed that his motivation, in part, came from not wanting to be part of the previous Soviet system. He said, “I think this is why many who came of age after the collapse of the Soviet Union are so driven.”1 It is true that Sukhotin and other like-minded Russian entrepreneurs have raised the bar in terms of product offerings in the foreign exchange market. And many FX brokerages have used Russian-inspired technology. Saxo Bank outsourced work to St. Petersburg-based Reksoft from 2003 to 2006. MF Global used Russian developers from Rostov to work on its retail platform—a hybrid of VT Trader, also used by CMS. Before moving its headquarters to Limassol in Cyprus, MetaQuotes found its origins in Kazan, Russia. Then of course there are the Russians who either founded brokerages or hold high-ranking positions in them. Alpari’s founders, Andrey Dashin, Andrey Vedhikin, and Gleb Petrov, all hail from Russia. As does FxPro’s Denis Sukhotin. Eduard Yusupov has been the global head of dealing for FXCM since 1999. With very few exceptions, almost all retail FX brokers offer MetaQuotes products and therefore have an association with Russian-built software.
But how was it that MetaQuotes extended its reach when on a relative basis, brokers using their own software simply stood still? The answer for the most part is found in the question! In the late 1990s several brokers launched retail FX using their own software. The intellectual property rights belonged to them, and they were constrained by the capital they invested. At the same time, profits from retail trading were enormous given the spreads involved, the frequent use of B Booking clients, and the propensity of novice retail traders to blow up. Brokers were motivated by greed and dollar signs, and far from developing white label solutions to quickly accumulate worldwide client bases as part of a parallel business strategy, many were content to gather quick and easy profits in their own backyards. Retail brokers were relatively small and comparatively understaffed.
Some early versions of platforms were clunky and not so user-friendly. White label solutions did evolve, but generally they involved a not insignificant profit share between the enabling party for all the associated add-on services necessary in providing the white label. The B Book also remained and belonged fairly and squarely to the broker offering the white label solution! The demand for retail FX far outweighed the supply of platforms scalable enough to handle the business. Trust was also an issue. Smaller brokers in less heavily regulated jurisdictions and with smaller capital bases wanted to offer FX and profit from it without the punitive tie-ups and risks to partnering with marginally better known FX start-ups.
Fatkhullin spotted an opportunity, and he had several competitive advantages that quickly led to him and MetaQuotes gaining a global footprint. First, he had a highly intellectual and like-minded workforce on his doorstep. Second, his cost of labor was not high. If he could develop a scalable, inexpensive, user-friendly platform, then he could issue multiple instances of it for a one-off license fee and a monthly recurring commitment fee. His staff members were galvanized by his vision. It provided a quick, relatively cheap, and viable alternative to brokers wishing to benefit from their client bases and also wishing to manage their own risk. Crucially, it also allowed clients to sign up with brokers they might be more comfortable with rather than sending their money offshore. Fatkhullin and his team had created a company with a product that generated an excellent positive monthly cash flow and that would grow incrementally with the number of licenses they sold. He absolved himself of any risk taking and from paying for expensive marketing and simply focused on something that he did best: computer software development. At the same time he delivered to brokers and clients an outstanding FX platform.
The entwined stories of MetaQuotes and Alpari took a leap forward in 2005. MetaQuotes had progressively been improving its software and applications for the previous four years, upgrading its MetaTrader platform, and it launched a trading platform for use on Palm devices. Alpari celebrated attracting its 10,000th customer in March 2005. By then, MetaQuotes had reached out to many hundreds of thousands of clients worldwide through its network of subscribing brokers. July 1, 2005, marked the official release of MetaTrader MT4, by far MetaQuotes’ most advanced platform to date. For one thing, it could offer FX, CFDs, and futures on the same platform. In addition, the platform was much more reliable and secure and able to efficiently resist “net attacks.” The client graphical user interface was more functional, and on the back-office side, additional tools were provided to brokers to process trades. In short, it was a vast improvement on the previous version of MetaTrader. What’s more, and momentous to the chronology of social trading, is that the platform also featured MQL4-IDE—a development environment for creating trading strategies. This was a tool that would help transition automated trading strategies and ultimately social trading from an inchoate idea to something of enormous magnitude.
SOCIAL TRADING
The adjective “social” has been popularized in this millennium, especially since the advent of companies such as Facebook, Twitter, and LinkedIn. Facebook has more followers than many religions, and it has added WhatsApp and Instagram to its suite of offerings. For its owners, it has become a cash cow with earnings before interest, taxes, depreciation, and amortization (EBITDA) at well over $6 billion at the time of this writing. The company joined the Fortune 500 list of companies by revenues earned in May 2013, and its founder Mark Zuckerberg is worth many billions of dollars.
Zuckerberg’s rise to stardom was swift, as was his company’s. Facebook was launched in February 2004 as an Ivy League networking website. By 2006, it opened up to anyone over 13 years old with a valid e-mail address. By 2007 it had attained a theoretical paper value of $15 billion when Microsoft acquired 1.6 percent of the company for $240 million, and by 2010 it had half a billion users. In April 2012, Facebook bought Instagram for $1 billion, and in February 2014, it bought WhatsApp for $19 billion. By August 2015, it had eclipsed 1 billion users, and its market capitalization topped $300 billion by November 2015.
While the concept of social media equates to massive marketing opportunities and Internet boom types of valuations for Facebook and others, the application of shared networks has not been lost on the online trading fraternity. It is perhaps due to social media that the term social trading came about. The benefits to marketing are relatively straightforward and clear vis-à-vis social media networks. Sample populations with similar characteristics can be segregated and targeted with any number of promotions. The advantages of social trading are less clear. But first we need a better understanding of what social trading is and how it came about.
In all walks of life, there are leaders and followers. In the financial markets, people’s spirits and wealth are generally buoyed by advancing equity markets. Positive equity markets tend to mean that companies are doing well, and so job security (or the chance of finding a job) is that much better. People can borrow more and spend more. People are more content and sanguine and feel far more reassured about their investments and also when they listen to and watch bull market experts opine effusively about the favorable aspects of holding stocks and the potential upward trajectory of the various stock indexes. It beats listening to bear market naysayers who predict imminent doom and catastrophe!
This cognitive human fallacy is known as the optimism bias or positivity illusion. The optimism bias holds true in declining markets as people tend to hold on to losing positions far longer than they should by somehow thinking that (a) they know better than the market or (b) they know better than their peers. Amos Tversky and Daniel Kahneman have put forward a theory in behavioral economics known as the “prospect theory.” They contend that the pain of loss in terms of utility far exceeds the equivalent pleasure of winning a similar amount. Humans will go to great lengths to avoid feeling pain. The theory explains, in part, why humans cut winnings quickly and run losses—something not lost on the many retail FX brokerages that run B Books.
The optimism bias is the reason that many people follow financial market gurus. There is a high chance that those gurus became gurus due to “survivorship bias.” (They outlived other gurus who got something wrong just that little bit earlier.) Think of a roulette table and watching somebody who correctly predicts either black or red successfully several times over. He might put all his chips in for the next spin of the wheel. The optimism bias might mean that you back him and you put your own money on “the survivor” to win. As is true of a lot of financial market gurus, he’s only as good as his luck. He is just as likely to get it right as he is to get it wrong. You either double your money in this example or your chips disappear with his.
Financial gurus tend to crop up in rising or bull markets. In Hong Kong, there was one revered guru who had such a following that crowds of people would pay significant entrance fees to attend his seminars. They would listen most attentively and hang on to his every word; they would applaud him rapturously; and some might even ask for his autograph at the end of his sessions. He was an older, bespectacled, not unintelligent man who said the right things at the right time, and he appealed to a great many people. He was a great one-man marketing machine, and no doubt he did very well from his local infamy. His candle fizzled out in the year 2008 as the global financial crisis hit worldwide equity markets.
The point here is not to deny this man his luck but to point out how many small lucky gurus could and still can monetize their luck without going to the great expense of setting up hedge funds. The answer comes in two forms: (1) trading for another person or persons with a limited power of attorney (LPOA) and (2) using either a percentage allocation module management (PAMM) structure or a multi-account manager (MAM) structure to allocate trades. Trading with an LPOA and either a PAMM or a MAM structure is where social trading traces its origins.
TRADING WITH A LIMITED POWER OF ATTORNEY
Allowing somebody to trade with a limited power of attorney (LPOA) on your account essentially means that you delegate trading authority to somebody else. The “limited power” part of the agreement generally stipulates that your trading agent cannot withdraw your money, but in most other respects, he has complete control over your account and certainly with respect to trading decisions. Before entering into an LPOA agreement with anybody, it would pay to be totally sure that the individual to whom you entrust your money is of the highest caliber and integrity. It would probably pay to have references from other people who have utilized his services.
You might also wish to check out which broker the trader uses. The temptation of some traders who trade with LPOAs is to “overtrade.” You may well have agreed on some performance incentive with the trader to pay her when you make money. The chances are, she gets a kickback from the broker from trades she executes on your behalf, and if she trades frequently, these hidden rebates can quickly add up. Performance can soon become secondary to the spread kickbacks she receives.
PAMM AND MAM ACCOUNTS
A progression of limited power of attorney comes with percentage allocation module management (PAMM) or multi-account manager (MAM) accounts. If two or more people give limited power of attorney to a money manager, he might use either a PAMM or a MAM structure to trade the accounts. PAMM is quite straightforward. Say the manager has a pool of funds from four different investors. When the manager executes a trade, then the trade is automatically split in the proportion that each investor holds in the pool.
For example, a pool of funds comprises $100,000:
Investor 1: $25,000 (25 percent)
Investor 2: $10,000 (10 percent)
Investor 3: $50,000 (50 percent)
Investor 4: $15,000 (15 percent)
If the investment manager were to buy EUR 1 million against dollars, it would be split as follows:
Investor 1: EUR 250,000
Investor 2: EUR 100,000
Investor 3: EUR 500,000
Investor 4: EUR 150,000
The advantage for the money manager is that he can trade a group of accounts as a single account. Clients will all receive the same execution price for the products traded, and profits and losses will automatically be allocated to each subaccount based on each account’s share of the total pool. Any agreed commissions are automatically credited to the money manager’s PAMM account. If a client were to withdraw his funds or a new client were to join the pool, the percentage allocation would alter according to the new pool of funds and the relative percentages of each investor.
Many money managers prefer to use MAM structures for their additional flexibility in trade allocation. MAM structures are typically used on MetaTrader, and they allow a manager to execute block tradesand allocate those trades into subaccounts according to different parameters. Whereas PAMM structures allocate trades according to the size of the pool of funds, MAM structures can allocate trades in ratios according to a specified number of lots (one lot is typically 100,000 units of currency), a percentage allocation model (like a PAMM), or according to the leveraged position requested by the investor. They also allow for partial closeouts (again required for highly leveraged investors) so that the overall strategy is not disrupted by any single investor.
Things have come a long way from the days when a money manager was trading with LPOAs for a few accounts. MAM accounts can quickly distribute trades from managers using Expert Advisors (robots) and can service hundreds of trades across hundreds of accounts almost seamlessly. They are MT4 and MT5 brokers’ sources of untold riches and a great source of income for successful money managers. Some clients do quite well, others less well. Needless to say, it makes MetaTrader a very sought after platform.
As with Facebook, 2006 saw a great many opportunities open up for MetaQuotes, and MT4 brokers started to pop up across the globe. Alpari, its original backer, gained licenses to offer FX and CFD trading in the United Kingdom. FXDD, another early adopter of MetaTrader, offered its products out of the United States. Avatrade saw its beginnings in Dublin. FxPro set up as EuroOrient Securities & Financial Services, Ltd., in Cyprus. GO Markets opened its doors in Melbourne, Australia.
THE SUCCESS OF EXPERT ADVISORS
MetaQuotes had a coup in December 2006 when it organized its first ever Automated Trading Competition (Table 7.1). There were 258 developers of Expert Advisors who competed in the 12-week trading contest for a prize fund of $80,000. The winner, Roman Zamozhniy, multiplied his starting “virtual cash” of $10,000 by 250 percent. The word was out, and trading robots were fast becoming a thing of “the now.” MetaQuotes would organize an Automated Trading Competition again in 2007, in 2008, 2010, 2011, and 2012.
TABLE 7.1
MetaQuotes Automated Trading Championships Results
MetaQuotes Automated Trading Championships Results

The year 2008 saw over 700 competitors vie for the top prize of $40,000. The panel of judges included Renat Fatkhullin and representatives from the online retail brokerages FXCM, Interbank FX, and FXDD. These companies would sponsor first, second, and third prizes.
The winner that year was Kiril Kartuniv of Bulgaria, who turned a virtual $10,000 into just under $170,000, exceeding the previous year’s winning total of just over $130,000. The year 2010 saw a winning total of $77,102; 2011, a total of $113,115; and 2012, a little under $55,000. Impressive trading indeed, and magnificent headline results. Plenty for retail brokerages to shout about. But as ever, there was a twist. A deeper analysis of the results revealed that less than a third of the Expert Advisors in any of the competitions were profitable. In fact, in 2006, 2007, 2008, and 2011, fewer than 20 percent of the systems were profitable!
It is an admirable achievement that Renat Fatkhullin has created a medium for trading algorithms to operate on his platform. It is not really for him to monitor the ineptitude of some of the robots. Suffice to say if only 21 percent of robots make money, then 79 percent lose money. (In the 2006 competition, the losing robots lost five times more than the winners gained.) In 2010 (the robots’ best year with 32 percent profitable), the losing robots still lost more than the winners. In 2012, it was the same story. The odds would appear stacked against anyone who wishes to follow a robot! Add slightly wider spreads, and the odds of winning diminish further—something, it has to be said, that is not lost on retail brokerages running B Books.
B BOOKS AND THE STRAIGHT-THROUGH PROCESS
Licensing MT4 or MT5 and offering a suite of Expert Advisor trading algos that clients may follow while simultaneously running a B Book might seem a little like a conflict of interest when it is statistically very likely that clients will lose. And yet it is the modus operandi of many brokerages that offer MT4 and MT5. Other brokers may offer a more palatable straight-through process (STP) modelwhereby they charge a slightly higher spread between bid and offer and pass the risk of each particular trade to the bank or non-bank provider that pledged the quote.
There are merits to both systems of course. As Alpari, FXCM, and many other brokers found to their cost, when a trade is passed straight through to a counterparty, the client’s position is held in the broker’s name at the client’s prime broker. If the client cannot pay her margin call, it is the broker’s capital that needs to support that trade and any potential losses.
A comparison of a B Book versus a straight-through process is as follows. In this example, a client trades 1 million EUR/USD each with two different brokers and executes a buy trade followed by a sell trade. First, I cover the B Book methodology.
The B Book Process
There are only two parties involved, a client and a broker:
Client ↔ Broker
EUR/USD quote 1.10201/1.10213.
The client buys 1 million EUR at 1.10213. The client is long 1 million EUR; the broker is short 1 million EUR at 1.10213. Let’s say the euro goes lower. The client sells 1 million EUR at 1.10013. The broker buys them from him. Both the client and the broker are square.
The client has lost 1.10213 − 1.100013, or 0.0020 or 20 pips × 1 million = $2,000. The broker has the other side of the trade and so therefore makes $2,000. If a broker holds hundreds of small accounts that are highly leveraged, the odds are that the broker will make a fortune as clients fail. Most clients will blow up by themselves. The evidence of robotic trading is that trading algos will also cause over 70 percent of clients to lose money.
The Straight-Through Process
Client → Broker → Price provider → Give up to broker’s account at prime broker
In this instance, the broker adds a spread. The broker sees the quote as 1.10201/1.10213. The broker quotes the client a slightly wider euro spread. The EUR/USD quoted by the broker is 1.1020/1.1022. The client buys EUR at 1.1022 from the broker.
The broker buys EUR at 1.10213 from the price provider. The price provider gives up the trade to the broker’s prime broker. The broker’s electronic platform sends a corresponding trade to the broker’s prime broker. The broker’s prime broker matches the two trades: the broker versus the client and the broker versus the price provider. The broker’s profit is 1.1022 − 1.10213, or 0.00007, or $70.
The Two Models Compared
The STP process is repeated on the sell side. The broker earns only the difference in spread. It appears to be a fairer system because the broker doesn’t profit (or lose) by taking the other side of the client’s trade (as happens in the B Book trade). In theory, it makes for a safer system for protecting the client’s money because if all clients win, then a broker running a B Book model is vulnerable to losing his capital. Counterflip the scenario: if the clients lose, the broker collects the spoils. STP works very favorably for brokers if clients trade frequently. Spread capture is also magnified by leverage.
Paradoxically, STP as a system failed in January 2015, when the Swiss National Bank (SNB) unexpectedly unpegged the Swiss franc against the euro, causing several brokers to incur huge losses and subsequently file for bankruptcy. Customers holding long EUR/CHF positions and employing huge amounts of leverage on small cash positions exposed themselves and their brokers to catastrophic losses. The flash crash in EUR/CHF that followed the unpegging meant that stop-losses were filled several hundred pips from where they were left, resulting in many small retail investors facing margin calls in the millions of dollars.
It became clear very quickly that it would be impossible for some clients to meet these calls, and so many brokers in turn were left with massive margin calls at their prime brokers. While clearly it brought the dangers of excessive leverage to light, it also raised questions about which systems brokers should use to mitigate their risks. Ironically, those running B Books did not lose in this scenario. However morally abhorrent B Booking may be to some who compare retail brokers to casinos in terms of how they operate, it is actually the brokers who employed this model who suffered the least from the fallout in EUR/CHF.
The Swiss National Bank debacle has little to do with MetaQuotes save by association to FXCM and Alpari, two of its largest supporters that were both dealt grievous blows with the fallout of the Swiss franc. Alpari and FXCM ran straight-through processes, and both took crippling hits. Alpari filed for bankruptcy. FXCM took a large loan at a punitive interest rate to stave off the liquidators, and it managed to stay in business. Some people may level the blame at high-frequency traders for the rapidity and exaggerated move in the many Swiss franc currency pairs. But the HFTs in question were more of the institutional kind. The robots that trade on MetaTrader, in general, are not of the high-frequency variety. They have been developed by programmers to follow any number of trading signals and indicators, short-term oscillators being the favored kind.
THE DANGERS OF USING EXCESSIVE LEVERAGE
It must be reiterated at this stage that trading FX is effectively a zero-sum game. That means that the odds of winning are 50:50 in a market with no spreads. So if spreads are involved, the odds of winning are less than 50 percent. Those who advocate trend following tend to follow longer-term trend following strategies employing sensible amounts of leverage. A sensible amount of leverage is between 2 and 4 times capital. An amount of 10 timesleverage is high; 20 times is considered very risky; and 100 times means a drawdown of 1 percent, and capital will be erased. At 400-to-1 leverage, capital will be wiped out with a quarter percent move in the wrong direction. If spreads are involved, capital will erode that much more quickly when compounded by leverage.
A secondary point is about time frames. There are many of them. Traditionally traders might use hourly, four-hourly, daily, weekly, and monthly charts in their analysis. Nowadays, there are a lot of systems that trade on minute charts, 5 minutes, 15 minutes, and hourly. Some might argue that patterns can evolve in any time series. Others might counter this and say that more frequent trading allows for collection of more spread and commission earnings.
In the realm of Expert Advisor trading algorithms, there are many that employ short-term high-turnover models. It is extremely difficult to identify what, if any, competitive advantage these systems have. Some report incredible returns in the thousands of percent. A 1 percent favorable move leveraged 400 times does equate to 400 percent after all. And a 3 percent move therefore would equate to 1,200 percent. These are the kinds of headline returns that entice inexperienced traders to follow these systems, and in many cases they pay for the privilege.
TRACKING SOCIAL TRADING PERFORMANCE
Transparency is one of the aspects of social trading that win it enthusiasts. MetaQuotes technology allows Expert Advisors to register their systems and have their performance tracked. It allows traders to subscribe and follow some trading robots for free or by paying others a monthly fee. The fees are generally in the range of $20 to $100 per month. There are lots of transparent statistics about each trading signal. The headline information statistics are the name of the system, monthly subscription cost, percentage growth, how many subscribers, how many weeks the system has been running, how many trades, win percentage, and profit factor. A typical headline figure may appear as shown in Figure 7.1.
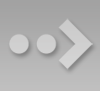
Figure 7.1 Trading robot typical headline.
Drill down into the system, and you will find many more interesting statistics. These include account initial balance, current balance, leverage employed, best trade, worst trade, gross profit and gross loss, winning trades, and losing trades (Figure 7.2).
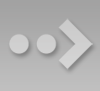
Figure 7.2 Trading robot typical statistics listing.
In the example in Figures 7.1 and 7.2, there are some telltale signs that the headline 6,500 percent growth is extremely vulnerable and that investors wishing to subscribe for $30 per month may expose themselves and their capital to a reversal of fortune in performance:
First, the level of leverage is frightening.
Second, the system has been up and running for only 12 weeks.
Third, while winning trades exceed losing trades by 2 times (601 compared to 300), the average losing trade at $176 exceeds the average profitable trade of $110 by a factor of 1.6 times.
Fourth, the worst ever trade of $803 exceeds the best ever trade of $601.
Fifth, this account started with only $200 and currently has only $13,200.
In summary, a rather cynical opinion of this hypothetical Expert Advisor is that it is a highly leveraged churning algo with poor risk management. Its performance is probably due to luck. The equity in the account is minimal, and the trades are unlikely to be scalable in any reasonable size. It might be quite fun to throw $100 at it to see if you can double your money, but you certainly should not invest $1 million. There, in a nutshell, really lies the problem with following trading algos. They are not built for scale! There are no doubt some very good (or lucky) algos out there that have produced astronomical results. But ask the question, “Can they do this in any scale?” and the answer is a categorical no!
To better explain my point, I will give a hypothetical example. Let’s say we have a short-term trading signal that buys and sells on meandering trendless markets. Perfect for an algo built to trade on a combination of time and change of direction. Let’s say the algo uses a 5-minute chart and a 15-minute chart and the system buys or sells when there is a crossover of a moving average on these two charts. Let’s also say the system is designed to take a 10 pip profit and stop itself out on a 5 pip loss.
This very basic trading algo no doubt could be refined to add other parameters to enhance its performance. The algo might work stunningly well. There’s no reason to say it shouldn’t on a small amount of capital and on a liquid currency pair. So with $1,000 invested and 200 times leverage, it can quite easily trade USD/JPY $200,000 without having any effect on the market. At $10,000 invested, it’s quite likely that there will be no material diminution of performance as $2 million USD/JPY will be quite easily absorbed into the market.
At amounts above $2 million and certainly above $5 million, the best available bid and offer prices are distorted and will start to widen. So with capital of just $25,000 employed in this trade, performance starts to trail off. This is certainly not the fault of the Expert Advisor, nor is it the fault of the broker who provides the price. It certainly has nothing to do with MetaQuotes or its platform. It is, however, a reality of the market. As trade size gets bigger, market makers need to widen their price to cover their risk. If we elaborate this example a bit more, we can add HFTs to the mix, and as explained in the previous chapter, the effects of “spinning the stack” by HFTs come into play (Figures 7.3 and 7.4). With just $500,000 invested, the system has the ability to trade $100 million. (This could be $200 million at 400 times leverage.)
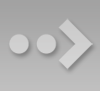
Figure 7.3 Liquidity stack. The first $2 million can be covered at 120.212. To buy $100 million, the price for the last $30 million is 120.27.

Figure 7.4 The effects of “spinning the stack” by HFTs. Here you buy the last $30 million at 120.28.
Notice that the first $2 million can be covered at 120.212 and that to buy the whole $100 million, the price for the last $30 million is 120.27 (Figure 7.3). The volume-weighted average price (VWAP) should be 120.2472. This is 3.52 pips away from the buy signal. HFTs distort this price further. Once HFTs are involved, the last $30 million is covered at 120.28. The VWAP is 120.2568. There is slippage of 4.48 pips. (Figure 7.4)
If this trading algo were to manage $1 million and trade $200 million, it is fairly unlikely that it would be able to buy this amount without 10 points slippage from the best-offer price of 120.212. The system cannot function because if the take profit were 10 pips away from the initial entry price, it would be slipped on the sell side too. It would end up losing more than 10 points in slippage against a 10 point profit objective. It is therefore a system that effectively creates a profitable trade but cannot trade it in any decent size whatsoever. Performance of algos with capital of just $50,000 or more will be impaired if they trade on short time frames with high leverage. If 79 percent of over 2,700 algos employed in six MetaQuotes trading competitions lost money with starting capital of just $10,000, it is most unlikely that anybody will become a currency millionaire, let alone a billionaire, by following one of these systems!
ZULUTRADE
The first Automated Trading Competition held in 2006 turned out to be a spark for an explosion in Expert Advisor development and in companies offering automated trading via PAMM and MAM structures. One such company that formed in Virginia in the United States in 2007 was ZuluTrade. Its founders, Greek entrepreneurs Leon Yohai and Kostas Eleftheriou, subsequently set up their headquarters in Athens, Greece. ZuluTrade is connected to 64 brokerages, and it offers what it calls a “peer-to-peer service.” ZuluTrade predates the Expert Advisor subscriber and follower model of MetaQuotes, and in many ways it inspired MetaQuotes to set up its MQL5.com website and Trading Signals service, whereby investors can select and pay to follow Expert Advisor trading algos.
How it works with ZuluTrade is that in principle, ZuluTrade acts as an introducing broker to the 64 brokers it works with. If an individual wishes to subscribe to one of the Expert Advisors (EAs) that ZuluTrade promotes, he may do so. The client will then sign an agreement with the broker with whom he has an account to agree and acknowledge that a “pip rebate” goes back to ZuluTrade, which then disburses a proportion of that pip rebate to its Expert Advisors. In many ways this is a much more expensive way to trade than following an EA through the MetaQuotes route. The EAs on MetaQuotes charge a monthly fee. On ZuluTrade, a commission is paid on every trade. It might not hurt, but it certainly pinches! And if trading is frequent, many pinches cause severe bruising to profits. What is also striking about some of the headline figures for EAs profiled on ZuluTrade is that they trade in single lots or fractions of lots. A single lot is equivalent to 100,000 currency units. A master account that trades in single lots will necessarily have a better performance than a slave or follower account that trades in larger quantities, pays a commission, and is subject to slippage.
METATRADER’S POPULARITY
Part of the reason for MetaTrader’s popularity is the ease with which it can be licensed and its ability to run many thousands of trading algorithms. A properly marketed successful trading algorithm can bring thousands of dollars in monthly fees and possible spread rebates to its successful developer. This fact has not been lost on many companies that set up as “algo libraries.” It is not lost on MetaQuotes either. There are many firms competing in the race to monetize this aspect of social trading. Companies such as Collective2, which offers 56,000 strategies developed by 18,000 traders whom you can follow at $99 per month, is one such example. To give credit to Collective2, they are a company that does give an explicit warning about following trading strategies. It reads as follows:
Past results are not necessarily indicative of future results.
These results are based on simulated or hypothetical performance results that have certain inherent limitations. Unlike the results shown in an actual performance record, these results do not represent actual trading. Also, because these trades have not actually been executed, these results may have under- or over-compensated for the impact, if any, of certain market factors, such as lack of liquidity. Simulated or hypothetical trading programs in general are also subject to the fact that they are designed with the benefit of hindsight. No representation is being made that any account will or is likely to achieve profits or losses similar to these being shown.
In addition, hypothetical trading does not involve financial risk, and no hypothetical trading record can completely account for the impact of financial risk in actual trading. For example, the ability to withstand losses or to adhere to a particular trading program in spite of trading losses are material points which can also adversely affect actual trading results. There are numerous other factors related to the markets in general or to the implementation of any specific trading program that cannot be fully accounted for in the preparation of hypothetical performance results and all of which can adversely affect actual trading results.
It’s the same old story: performance dwindles with scale. In this case “lack of liquidity” could be defined as wider spreads associated with trading larger amounts or trading in less liquid currency pairs. Also, there are “numerous other factors.” It is interesting that Collective2 implies that in some cases traders might not “adhere” to a particular trading program in spite of trading losses. In other words, traders are human and the human element might “override” the system. Optimism bias takes over!
Other variants on the same expert trader-advisor theme are “educators” and their websites. An FX education website, normally run by an ex-FX trader or expert, may boast of a superlative trading approach, may tutor on “risk management,” and then might offer some kind of a hybrid oscillator with several entry, take-profit, and stop-loss levels, the high number of which will ensure daily activity in the market. The educator makes money in two ways: (1) she charges for her system, and (2) she links her website to preferred brokers. She will receive some kind of compensation when clients referred from her website sign up and trade with her preferred brokers.
Some innovative companies, such as Quantopian, employ a merit-worthy community-based approach whereby developers of trading code can communicate on a blog, and once they have developed their own algos, they can enter them in a monthly competition. It is not too dissimilar to MetaQuotes with its MQL5 community and the Automated Trading Championships. The differentiating factor is that Quantopian aims to back the best algorithms and find investor capital for them to trade in what it describes as a “crowd-sourced hedge fund.” Quantopian will provide the capital, technology, legal, and compliance operations. The top programmers will provide the investment strategies. Quantopian will match top-performing managers with outside investor capital to fund their ideas. Quantopian uses Python as its coding language and supports Robinhood and Interactive Brokers.
With the many thousands of programmers and the abundance of incredible brain power working at beating the market, the chances are that there are some very good traders and Expert Advisors. Ditto FX has endeavored to harness some of what it considers to be “top managers with demonstrable track records,” and it offers out their services for a connecting fee in what amounts to a similar model to other, more traditional algo libraries. Run by a successful former arbitrage trader, the company focuses on FX fund managers with at least $5 million under management. Track records run into years rather than weeks and months. Returns are real and not hypothetical. They are also attainable and sustainable rather than unbelievable and improbable. Typically its traders and their systems employ leverage of under three times, which in some ways explains why they have a chance of staying in the game. Naturally, they use MetaTrader MT4!
THE VALUATION OF METAQUOTES
Inevitably, we arrive back at MetaQuotes. In March 2010, MetaQuotes launched its MQL5 website for MQL5 application developers. The website had the explicit aim “to provide all visitors with an opportunity to freely communicate and discuss issues of programming in MetaQuotes Language 4 (MQL4) and MetaQuotes Language 5 (MQL5), trading, automated trading systems development, strategy testing, and using technical indicators in MetaTrader 4 and MetaTrader 5 platforms.”
In June 2010, MetaQuotes launched its Metatrader 5 platform for trading FX, CFDs, and equities on the world’s stock exchanges, and in October 2012, it launched Trading Signals, its own social trading network that can be accessed through both its MetaTrader4 website and the MQL5 website. There are already 800,000 MQL5.community users. It supports seven languages, and it offers over 7,000 trading applications, 4,000 Trading Signals for automated copy trading, and 6,200 free source codes. It provides access to 500 professional programmers who can be commissioned to create custom-made trading robots. The scale of MetaQuotes is immense relative to any of its industry peers. And it is growing!
It is difficult to put a value on MetaQuotes because of its massive potential worldwide. If Saxo Bank has 120 white label partners, then MetaQuotes has over 450 brokers who use the MQL platforms. If FXCM has 178,000 active clients, GAIN Capital 150,000, and CMC Markets 75,000, then MetaQuotes, through its partnerships has 7 million who use MetaTrader, of which over 1 million trade on mobile devices. If Collective2 offers 56,000 hypothetical strategies at a subscription charge of $99 per month, then MetaQuotes already offers 7,000 trading aps and 4,000 Trading Signals. It also has a following of 800,000 in its MQL5 community who can access 500 professional developers at any instance. On any metric you choose, MetaQuotes blows the competition away.
Of the retail brokers who have floated on the world stock markets, GAIN Capital as of February 2016 had a market capitalization of just over $330 million, at the low end of its range over the last five years. FXCM, similarly, is trading well below its $1 billion IPO valuation. CMC Markets floated on the London Stock Exchange in February 2016 with a value of GBP 691 million. At one stage, Australian broker Pepperstone was looking to float on the Australian ASX, with some pundits speculating that its valuation would be in the region of AUD$600 million. Pepperstone uses MetaTrader almost exclusively, and its main client base is in Asia Pacific. FxPro, another MT4 user, looked to float as early as 2011 with a valuation in excess of GBP 200 million. Alpari, which came unstuck in 2015 and was one of the original backers of MetaQuotes, missed out on an IPO. It claimed to have 100,000 clients as early as 2009.
It’s a well-known fact in the world of retail FX that rough estimations of earnings can be made from a computation of retail client equity balances and number of active clients.
We can therefore develop a simple heuristic company valuation based on number of active clients, net revenue per client, and some kind of industry multiple. So, for example, 20,000 active clients earning a company $2,000 each in net revenues and multiplied by an industry price/earnings (P/E) ratio of 12 equates to a valuation of $480 million (20,000 × $2,000 × 12). If 50,000 clients were earning a company $1,000 each in revenue at a P/E of 12, that would equate to a $600 million valuation. There are obviously many other factors that contribute to the value of a company, not the least of which is the potential growth and intellectual property. One can only hypothesize about a valuation for MetaQuotes if it stood as a stand-alone company and was the sole provider of its platform. It would be billions!
The tangible aspects of a valuation for MetaQuotes are that it generally charges $100,000 to license MT4, and thereafter it charges $1,500 per month for ongoing maintenance and $750 to support mobile applications. In addition, it receives 20 percent of monthly subscription rates for Expert Advisors. It has been suggested that MT5, its new platform, can be licensed for $500,000.
Currently there are 450 brokers who offer MT4. They would have already paid up $45 million.
So monthly fees are $2,250 × 450, or just over $1 million per month, or $12 million per year.
Let’s say they sell 50 new licenses per year for MT4 at $100,000, or $5 million.
And 20 MT5 licenses at $500,000, or $10 million.
Add revenues from aps, virtual hosting, and EA subscriptions and add, say, $3 million.
A guesstimate of $30 million doesn’t seem so much. But what is the potential? And you might well attribute a multiple of 50 to 100 times earnings. The reasons are principally as follows:
1. A large proportion of the retail trading FX community depends on MetaQuotes for its software. It has millions of users.
2. The same goes for the programming community.
3. MetaQuotes charges are not high. They could be higher!
4. As yet MT5 is not the platform of choice. There may be a point in the future when MetaTrader MT4 is phased out and MT5 becomes the de facto trading platform for the MetaQuotes community.
5. There are 800,000 community members of MQL5.
6. MetaQuotes MT5 has passed conformance testing, and it has been certified on the Moscow Exchange, the Dubai Gold and Commodities Exchange, BM&FBOVESPA, Brazil’s largest exchange, the Chicago Mercantile Exchange, the Warsaw Stock Exchange in Poland, the Australian Securities Exchange, Borsa Istanbul in Turkey, the Pakistan Mercantile Exchange, the Stock Exchange of Thailand, and the South African Futures Exchange.
7. MetaQuotes has representative offices in Turkey, China, Singapore, Japan, Australia, Brazil, United Arab Emirates, South Africa, Pakistan, and Thailand.
The reach, depth, and scale of MetaQuotes and its products are phenomenal. Based on the above, MetaQuotes could easily be worth several billion dollars. It seems to have entered markets where others have failed to venture. It has penetrated many emerging market countries. It has a higher proportion of retail traders trading on its platform than any other single retail platform, and it also has a high proportion of programmers who apply their Expert Advisors to trade automatically on MetaTrader. Smaller brokers are dependent on MetaQuotes. Others base their IPOs on the popularity of MetaQuotes. Renat Fatkhullin and his team have very nearly monopolized the whole industry by being smart. And monopolists, ultimately, can charge what they like!
SOCIAL TRADING NETWORKS
Although there is very scant information circulating about Renat Fatkhullin and his past, the Kazan connection does run very deep. The cofounders of Alpari, Andrey Dashin and Andrey Vedhikin, were both raised in Kazan. Dashin graduated from the Kazan State Institute of Finance and Economics in 1996. Vedhikin graduated with distinction from the finance and economics unit of Kazan State University in 1997 prior to picking up a PhD in economics. Metaquotes Software Corp. set up its first office in Kazan in the year 2000. It is run by Lenar Fatkhullin, although there is no reference to him as being a relation of Renat. Alpari linked arms with MetaQuotes in 2000 and executed the first ever trade through its platform in November of that year.
Now it seems that Cyprus is the new hotspot for software developers, programmers, and brokers. Cyprus is home to at least 50 brokers and also to MetaQuotes. Most of the brokering community offers MT4 and hosts social trading facilities. Typical social trading innovations include blogs, information about social community trading positions (sentiment), other information concerning conglomerations of stop-loss orders and limits (open orders), and transparent details of the trading performance of any number of Expert Advisors, which can be followed.
It seems that in some ways, the real-time communication of thousands of smaller traders is an effective tool in the decision-making process of that community and complements more traditional technical and fundamental analysis. Social trading networks are also forums where trading clubs can band together and trade in the same direction. Or where gurus with sufficient clout can direct their followers to trade. There is a real possibility that a significant retail-based network could actually move markets! Retail trading makes up about 8 percent of the FX market, and so while small, it is still significant relative to the whole. And its weighting is likely to grow.
A TRULY INNOVATIVE AND VISIONARY ENTREPRENEUR
Renat Fatkhullin is a genius, perhaps matched only by Kim Fournais and Lars Seier Christensen at Saxo Bank in terms of what they have provided for the retail FX trading public. (Saxo Bank added its own variant of social trading, launching TradingFloor.com in 2014.) Fatkhullin has focused on delivering a low-cost, scalable platform to a worldwide network of brokers and added functionality to cater to multiple product types. His telling innovation was to enable thousands of programmers to create trading robots to trade using his software.
Like many of his generation, he had a vision, and his was to be a professional software developer in the field of foreign exchange. Not only did he create exceptional software, he also created a coding language applicable to his platform. He worked with similar like-minded entrepreneurs and was able to quickly deliver a market-leading product. Perhaps two of his competitive advantages were that he could work with many experts in a low-cost environment and deliver a state-of-the-art platform at the genesis of online retail trading. Another might be that he focused solely on software development and let others indirectly market the platform on his behalf. He has been incredibly fair in licensing his product, charging relatively little to brokers who can connect directly with their client bases. At the same time he has captured an enormous market share of the online retail trading community.
His eureka moment was the inception of the Automated Trading Championship, which has taken retail FX trading to new levels. Just 258 traders took part in the inaugural competition in 2006, which has acted as a catalyst for an explosion in the growth in retail foreign exchange trading. Now, millions of people can follow Trading Signals or copy trading from hundreds of thousands of trading algos and trade through hundreds of brokers all connected to MetaQuotes MT4 or MT5 trading technology. Brokers trying to emulate MetaQuotes have a toss-up between trying to develop their own software and simply joining the bandwagon and purchasing a license to offer MT4 or MT5 to their clients.
Several brokers have made astronomical profits using a combination of MT4, trading robots, and elevated levels of leverage and by running B Books to profit from some less successful trading algorithms and retail traders. Some have even considered floating on world stock markets on the back of their impressive gains. Fatkhullin steers clear of controversially acting in the dual capacity of software developer and bookmaker, preferring to focus on what he does best in simply delivering his product to an ever increasing number of brokers, banks, and exchanges and listening and adapting his platform to meet the requirements of his clientele.
In an ever evolving, more scrutinized market, MetaQuotes delivers what the public wants and what brokers want. All Trading Signals have transparent track records, and social trading communities are capable of informing, educating, and guiding each other to make appropriate trading decisions. If there is a lesson that the trading public and novice investors should learn, regulators can pass that lesson down through brokers. A wary public should be aware that headline gains of several hundred or several thousand percent are not sustainable in the long term. In order to achieve those types of gains in the short term, huge leverage is involved, and slippage in following such systems is highly likely as is deterioration of performance proportional with larger investments. MetaQuotes is an enabler of trading. It is neither a robot nor a broker. There are many good sustainable profit making robots. However, scalability should always be a question that anybody has in mind when following such a system.
Fatkhullin, like all good traders or innovative entrepreneurs, has kept himself low key and secretive. He has built his business organically and resisted the need to issue an IPO. If he were to do so, his company would be worth billions. Fatkhullin and MetaQuotes are to social trading what Zuckerberg and Facebook are to social networking. In the retail FX world, probably more than 80 percent of traders are touched by his influence. It is for that reason that it is an easy choice to name him Social Trading King.
Jim Simons is one of the richest men on the planet. The reason is his high performing and highly envied hedge fund, Medallion Fund, which has not realized negative returns since 1990. Jim Simon’ Medallion Fund is the best hedge fund Insider Monkey has come across. In 2007, Simons returned 73% and in 2008 he returned 80%. The return is exceptional as such incomparably high returns were realized during the time of the financial crisis. We believe if he had started investing 20 years before he did, he would be the richest man on this planet today.
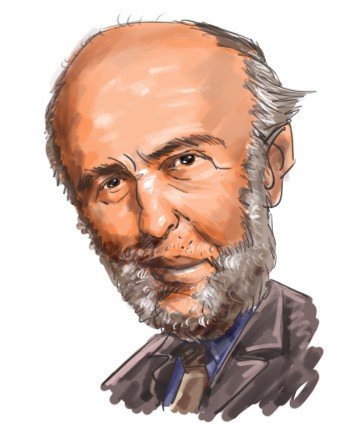
Jim Simons exploits market inefficiencies through high frequency trading. He makes use of algorithms to assist in execution of large orders. Consequentially, Medallion faces high expenses and transactions costs. This explains the higher than average performance fee of 44% that Medallion charges. However, the 44% fee is quite irrelevant considering the fact that the fund’s investors are current and past employees and their families. Jim Simons is the largest investor of Medallion and owns approximately 25-50 percent of Renaissance Technologies.
Jim Simons currently manages $24.85 billion. In the first quarter of 2011, Jim Simons reduced Lorillard Inc (LO) by 38%, Apple (AAPL) by 58%, Chipotle Mexican Grill (CMG) by 28%, and Google Inc (GOOG) by 27%. Since the end of March 2011, Lorillard Inc (LO) returned 12%, Apple (AAPL) returned 7.2%, and Chipotle Mexican Grill (CMG) gained 14.4% while Google Inc (GOOG) lost 1.3%. Here are Jim Simons’ top 20 stock positions at the end of March:
Johnson & Johnson (JNJ) makes up the largest portion of Jim Simons’ diversified portfolio. He increased JNJ by 21% in the quarter ending March 2011. He currently has 6.69 million shares of the company worth $396 million. JNJ returned 6.8% since the end of March. He has the largest position in the company after Warren Buffet. Warren Buffet’s Berkshire Hathaway has 42.62 million shares of JNJ, worth $2.5 billion. JNJ is among Warren Buffet’s top 10 holdings (See Buffet’s other bullish stocks).
In the first quarter of 2011, Jim Simons purchased Target Corp. As of now, he has 3.82 million shares of the company worth $191.4 million. Target returned -2.3% since March 2011. He has the largest position in Target Corp. The company makes up 0.77% of Simons’ current portfolio. Larry Robbins’ Glenview Capital has the second largest position. Robbins increased his Target holdings by 18% in the first quarter of 2011 (check out Larry Robbins’ top 20 stock holdings).
Lorillard (LO) returned 12% since the end of March. Jim Simons has the largest position in the company with 2.7 million shares valued at $256.7 million. Despite the decrease of Lorillard by 38% by Jim Simons in the first quarter, the company still makes up 1.03% of his portfolio. He also has the largest position in the company. Jean-Marie Eveillard’s First Eagle Investment Management has the second largest position with 2.29 million shares of Lorillard (see Jean-Marie Eveillard’s top stock picks).
Alcon Inc (ACL) was a merger arbitrage play which was acquired in April. Therefore, Alcon’s return is for only a few days. However, his remaining 19 stocks returned 0.3% since the end of March vs. a 9% loss for SPY. Jim Simons is very successful at picking winners this year. This is why he is beating the market. Keeping in mind his current and previous performance, we believe investors can beat the market by imitating his impressive stock picks.
No hay comentarios:
Publicar un comentario